How to identify product groups?
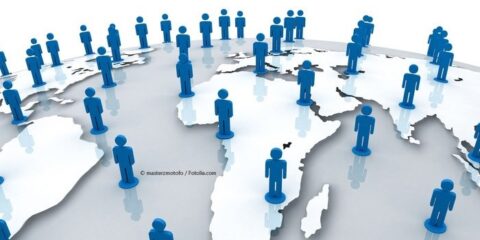
Ein Artikel von Dr. Christian Michael Schneider, ehemaliger Mitarbeiter DYMATRIX
Network Theory and its Business Implication – Part 3
This post is about the challenge of identifying product groups. Let us start with an example of a fashion retailer (without the loss of generality). The fashion retailer has a bunch of products in common product groups (male and female accessories, shirts, shoes, shorts and tops) and wants to know the optimal grouping in his online shop. Intuitively one would group the products into these ten product groups with two main groups (male and female) and then into a subgroup (accessories, shirts, shorts and tops). However, the other way round (first grouping by product and then by gender) is as intuitively as the first allocation.
At this point network theory comes into play. Instead of letting the retailer make a biased classification of the products into groups, one can use community detection algorithms based on transaction data to do the job. The underlying idea is the following: optimal grouping leads to groups which are never purchased together. Though usually it is not possible to get pure group purchases, the minimization of the number of cross-group purchases is sufficient to achieve good results.
One can start with customers’ market baskets and create a network with weighted links between products. The weighting is equal to the number of concurrent purchases as shown below on our retailer example:
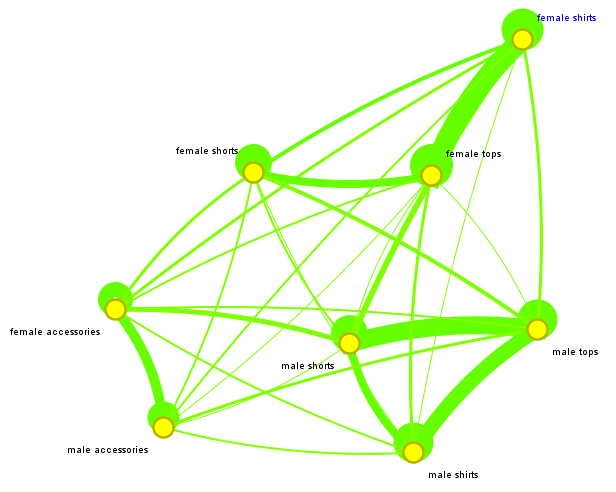
For this network the property „modularity“ can be calculated, which is related to the fraction of weighted group-links and weighted cross-group links. This modularity can be optimized with different algorithms, leading not only to optimal grouping, but also to hierarchical groups (for more details on community detection see [1]). Going back to the example the optimal grouping for the purest purchases for a particular algorithm is portioning the network into three groups (accessories, male, female) – and on the next hierarchical level into five groups (accessories, male-shorts, male-shirts+tops, female-shorts and female-shirts+tops):
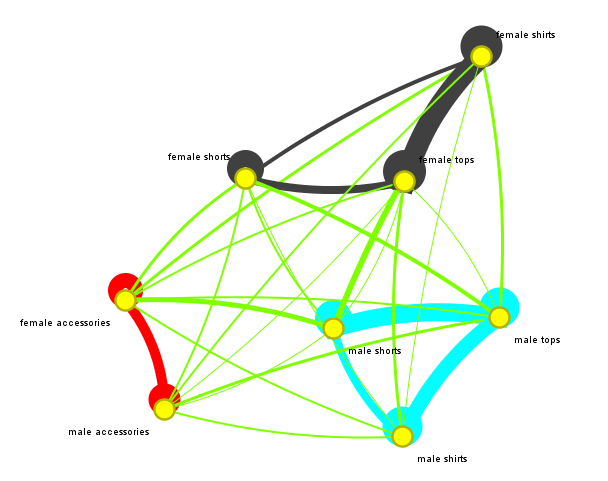
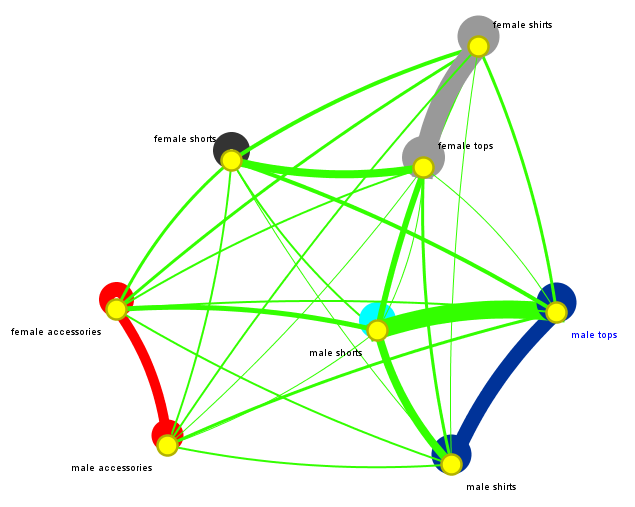
Now the Navigation bar of online shops should have three top-level tabs and two splits on the second level. Note that both number of hierarchy levels as well as number of groups in each level is not fixed in advance. This kind of grouping can also improve affinity models, since both predictors and targets are cleaner.
The next blog is about a happy and healthy company leading to happy customers.
Part 0. Network Theory and its Business Implication
Part 1. Identifying relevant business networks
Part 2. Is your customer or your customers’ friend more important for your business?
Part 3. How to identify product groups?
Part 4. How to keep your company happy and healthy?
Part 5. How to get customer (friendship) networks?
Part 6. Affinity modelling with network properties
[1] Community detection in graphs, Santo Fortunato, Physics Reports 486, 75-174, 2010