Automated Predictive Analytics – NBO, Uplift Models, Scorecards…
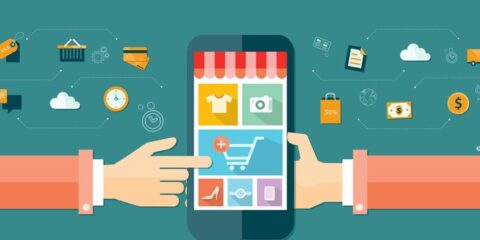
Ein Artikel von Erik Obermeier, ehemaliger Mitarbeiter DYMATRIX
As competition becomes tougher every day, it is essential to understand each and every customer’s individual needs – and to respond with the relevant offers. Predictive Analytics is the key to identifying customers’ requests.
However, how can you find the best possible answers to a growing number of questions without gobbling up ever more resources? And what options are there for businesses that lack in-house data mining experts but still want to run the very best prediction models?
I am convinced by fully automating the entire data mining process, you’d vastly reduce the resources required to maintain your models while preventing the downturn in effectiveness that usually comes over time. Your models – for a wide variety of questions, such as Next Best Offer, cross-selling and upselling, product affinity, potential optimization, churn prediction or forecasting (e.g. capacity utilization forecasting for your call center) – would be kept constantly up-to-date and therefore remain highly efficient, squeezing additional ROI by increasing the quality of your predictions. I.e. you can react swiftly to changes in customer behavior or in the market.
You could imagine 2 real life scenarios for a fully automated predictive analysis system:
- Businesses with Data Mining Experts: Experienced data analysts can actively intervene in processes when and as necessary. At the same time, the system relieves them of the mounting burden of everyday tasks such as monitoring, checking and updating existing scores – important but tedious work that seems to explode with each new mining model. The system manages efficiently some 1,000 models or more, freeing up precious time for the development of very complex models or new analytical scenarios.
- Businesses without in-house Data Mining Experts (Users without Data Mining Expertise): The system would enable even users without extensive data mining knowledge to quickly get started using analytical processes. The creation, maintenance and updating of models is fully automated, and the entire data mining process is standardized and automated.
Benefits are:
- Notable increase in prediction quality and ROI due to regular model evaluation
- Dramatic time savings in the creation, maintenance and quality control of data mining models
- Optimized campaigns: measurably more turnover due to continually updated customer scores and individualized product offers
- Ideal for use in complex environments: database preparation time is reduced to a minimum while integration into the campaign process is optimized (closed-loop marketing)
- Predictive analytics becomes a possibility even for non-experts
Such a system would have to offer functionalities as such:
- Framework for the automation of the entire data mining process, from data preparation to quality control of models
- Easy-to-use interface and straightforward management of numerous mining models
- Intelligent, self-learning software for the automated preparation, recalibration, updating and optimization of real-time scores
- Reports for illustrating current model quality and model trends over time
- Model Manager for managing and archiving models
- PMML compatible
- Standard interfaces to all common data mining engines, such as KNIME, SAS Enterprise Miner, IBM SPSS Modeler, MS Analysis Services, etc.
Therefore, you set the basis, but now we want to build on it and leverage it to beat the competition and serve our customer beating his highest expectations. You consider additional scenarios like:
- Uplift Modeler
An „Uplift Modeler“ makes use of the latest findings to create uplift effects. It allows you to predict the additional effects of direct marketing campaigns on customer behavior and to distinguish reactive customers from, say, those who don’t respond to contact, or those who might have become active even without contact. Ideal for cross-selling/upselling, churn prevention and customer retention. - Scorecard Builder
A „Scorecard Builder“ generates an easy-to-read scorecard from complex data mining models and allows the most important criteria to be highlighted, categorized and, if necessary, manually influenced. - Advanced Forecasting
An „Advanced Forecasting“ module allows you to predict numerous different volumes (e.g. inbound calls to Customer Service), employing elements of traditional time series analysis (autoregressive component) and correlations with associated time series (cross-prediction component). - Association Analytics
An „Association Analytics“ module automates the evaluation of complex shopping baskets (item-based NBO) and identifies anomalies (fraud detection). - Text Mining
A „Text Mining“ module uses, among others: semantic text mining, text filtering and standardization, definition of stop words and extraction of keywords, as well as trend recognition and classification as positive, neutral or negative.
Above, I covered the ideal world and I wondered for years whether such a system will eventually be available. I then learnt about DynaMine.
DynaMine is exactly the Predictive Analytics Automation system we are all looking for. It leverages any common data mining engines, such as KNIME, SAS Enterprise Miner, IBM SPSS Modeler, MS Analysis Services, etc. to such an extent that you wonder why it isn’t in use all over the place.